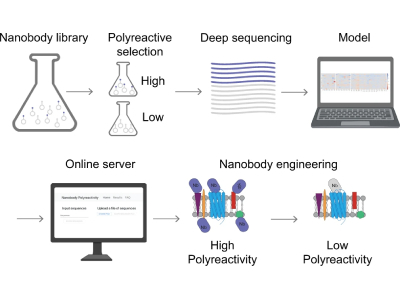
Credit: Edward P. Harvey et al.
Recent research from Harvard University utilized X-rays and machine learning to identify and predict features in antibody sequences that lead to polyreactivity. By predicting polyreactivity, researchers will be able to reduce the time it takes to identify potential antibody treatments as well as leading to the identification of those whose modification could lead to new treatments.
Antibody polyreactivity has long been of interest to scientists as it can lead antibodies to drift off and bind to the wrong molecules, presenting a liability in drug discovery and development. "Polyreactivity is the phenomenon where an antibody binds to a broad range of molecules that aren't its intended target," Andrew Kruse, a professor of Biological Chemistry and Molecular Pharmacology at Harvard Medical School said. "This is a common phenomenon for many antibodies. It's a major liability in drug development, and we would prefer to work with antibodies that are not polyreactive."
The study, published in Nature Communications, utilized the Advanced Photon Source (APS) at DOE's Argonne National Laboratory to identify highly highly polyreactive antibodies. To study individual antibody fragments the researchers utilized yeast display to isolate fragments and sort them based on polyreactivity. This separation and sorting allowed them to train a machine learning model to predict which features lead to polyreactivity, not only based on individual antibodies but their mutations as well.
When discussing the AT118 antibody, Kruse said "It binds to a variety of different things, and so we thought we could take that sequence, run it through our models, and try to predict mutations that would decrease its reactivity. We did that, and we came up with several mutations that lowered polyreactivity while preserving the antibody's ability to bind to its target. Then, we wanted to understand on a molecular mechanistic level how those mutations work. Why does this certain substitution change the reactivity properties of this antibody? To do that, we crystallized the antibody, we solved the structure at the APS, and we were able to see how these mutations actually changed the reactivity properties of this clone."
Kruse foresees future applications of the findings including utilizing these models to engineer antibodies to be less polyreactive or even identifying mutations that may make otherwise problematic antibodies more useful for treatments.