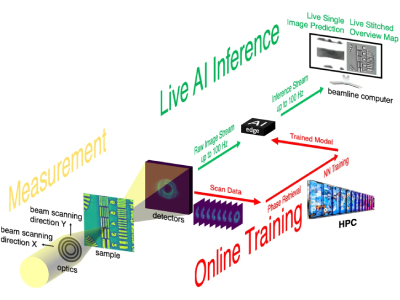
Illustration of AI-enabled workflow for real-time streaming ptychography imaging. Credit: Argonne National Laboratory
Researchers at the Argonne National Laboratory have developed a novel machine learning analysis technique to increase data processing speeds of x-ray microscopy research nearly 100-fold, while also reducing the amount of data collected. The increased efficiency will alleviate the data analysis bottleneck currently experienced by many researchers and could lead to an autonomous discovery system in the future.
“The problem is that conventional means of analysis can’t keep up with data rates,” said Mathew Cherukara, an Argonne computational scientist. “And so we’re in this situation where you have these amazingly complex, extraordinary pieces of hardware, but we don’t have a means of analyzing all the data that they can produce.”
Currently, x-ray microscopes are generating more data than can be efficiently analyzed, even with the help of supercomputing. While supercomputing reduces the time required from potentially weeks to just a few hours, the methods developed by the researchers at the Argonne National Laboratory further reduce the time required to a matter of minutes.
“Most people who come to the APS, they travel, they come prepared for a week of experiments, and at the end of the week, they leave with their data, which they would analyze back home,” said Tao Zhou an Argonne physicist. “If they find something interesting during the analysis that they want to do more measurements on, they typically have to wait for the next cycle of experiments at the APS. This technique essentially allows people to do the analysis in real time on the beamline, so if they see something new and interesting in the sample that they don’t anticipate, they can be ready and able to adapt almost immediately.”
The technique developed by the team, published in Nature Communications, is called streaming ptychography. The technique allows researchers, or machine learning agents, to make on-demand changes to the experiment in nearly real-time to focus on interesting phenomena or other observations.
“Having this embedded computing close to the beamline allows us to do on-the-fly adjustments immediately while the experiment is in progress without having to send data back to a cloud or supercomputing cluster,” said Anakha Babu, a former Argonne postdoctoral researcher. “This kind of setup can have uses beyond ptychography as well, in a wide range of experiments where adjustments based on the data can be needed in real time.”
The team also believes the machine learning method could be applied to other super-resolution microscopy techniques such as electron or optical microscopes to further advance and potentially automate discoveries.
“The most advanced microscopes in the world are no longer going to be held up by lack of analysis capabilities, and they’ll be able to operate at their full potential,” said Cherukara.