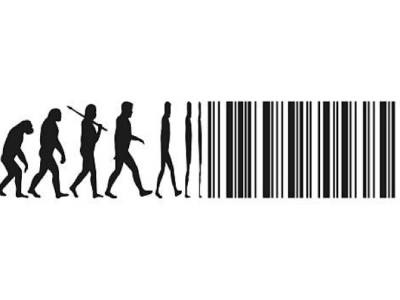
Data. It drives science, and it drives us. Discovery. It causes science to evolve. As scientists, we are driven by the next data point and how it will fit into some larger story that is progressing our scientific conversation. For over a score of years now, that conversation has involved our automation journey and, like science itself, our ever-changing attitudes toward data and how it’s used.
The Struggle for Existence
Automated liquid handlers (ALH) started to appear in life science laboratories by the dawn of the millennium. Rudimentary at the start, these devices improved data quality by removing pipetting variability from workflows and improving the repeatability and reproducibility of many laboratory procedures. ALH software and on-deck device hardware later advanced to permit scientists to increase the amount and complexity of the hands-free work that they were able to perform. Finally, assay-ready workstations emerged with decks that were pre-configured (i.e., incubator/shakers, thermal cyclers, etc.) and scripted methods pre-written for specific application kitted chemistries (i.e., sequencing by synthesis methods from Illumina). ALH technology quickly spread to every corner of the life sciences, and with good reason – data that was repeatable and reproducible.
However, data came at a price. Physics. The size of your ALH – and it’s work surface, or deck – directly determined (and limited) the amount of data that could be obtained. Moreover, ALH created workflow bottlenecks because many complex tasks needed to be taken off-line. Resulting modes of scientific thinking were similarly constrained by what was physically possible given the available deck size. ALH-centric automation limited our ability to adapt or grow, and the promise of sample-to-insight was never realized.
Variation
As the size and number of ALH proliferated, scientists realized that this mode of automation was not sustainable, and they searched for other ways to generate data. Many laboratory devices became smaller and more modular during this time which afforded scientists more opportunities to automate a wider range of functionalities, support any ALH devices they already had, and conserve lab space. Small modular devices outcompeted their larger predecessors as they became linked and integrated to mitigate procedural bottlenecks. Robotic arms were foundational to the success of these integration efforts, and early adopters were once again free to generate data and follow their science.
Survival of the Fittest
With the deck size paradox laid bare, and reliance on device flexibility and assay scheduling on the rise, the notion of achieving successful lab performance using singularly focused ALH-centric solutions was at an end. Fully integrated, and schedulable robotic systems were constructed by the likes of Paul Harper (AstraZeneca), Matt Sorge (Monsanto), and Daniel Tran (Genentech) that maintained gains in repeatability and reproducibility while offering better sample uniformity across robotic platforms and increased data production and output. What is more, the new systems were not limited to traditional areas of automation such as sample management or high throughput screening. Robot-based automated systems entered the drug discovery continuum (i.e., ADME Tox, QA/QC), proliferating to other application areas from consumer genetics to precision medicine. This disruption led to exponential increases in productivity, which was quickly realized. Scientists now wondered how to manage all the data.
Natural Selection
Laboratory automation tools now abound, and scientists use many types of solutions to nimbly adapt to changing requirements, choosing any device from any vendor to best suit their needs. Each automated workstation, and indeed every device integrated to it, generates scientific, meta-, and event-based data. Those who fully harness these data not only make better decisions faster – they drive the narratives within their scientific disciplines. More than improved metrics-based decisions, however, those who employ event-based data enjoy the additional benefits of maximized instrument productivity and full compliance with regulatory requirements. Companies like Recursion and others that combine data with innovative software, for example, have transformed the drug discovery space by improving their processes and, in turn, driving others to adapt as well. The result is unprecedented demand for software-based solutions that transform how data are managed.
We have come a long way from doing science at the laboratory bench. Today’s scientific discovery is driven collaboratively between human and robot within the life science data factory. Simple hardware solutions have evolved into interconnected networks of instrumentation and informatics which enable us to adapt and readapt to our changing scientific, technological, or organizational landscape at unprecedented scale – designing, building, testing, and collaborating with our colleagues wherever in the world they may be.
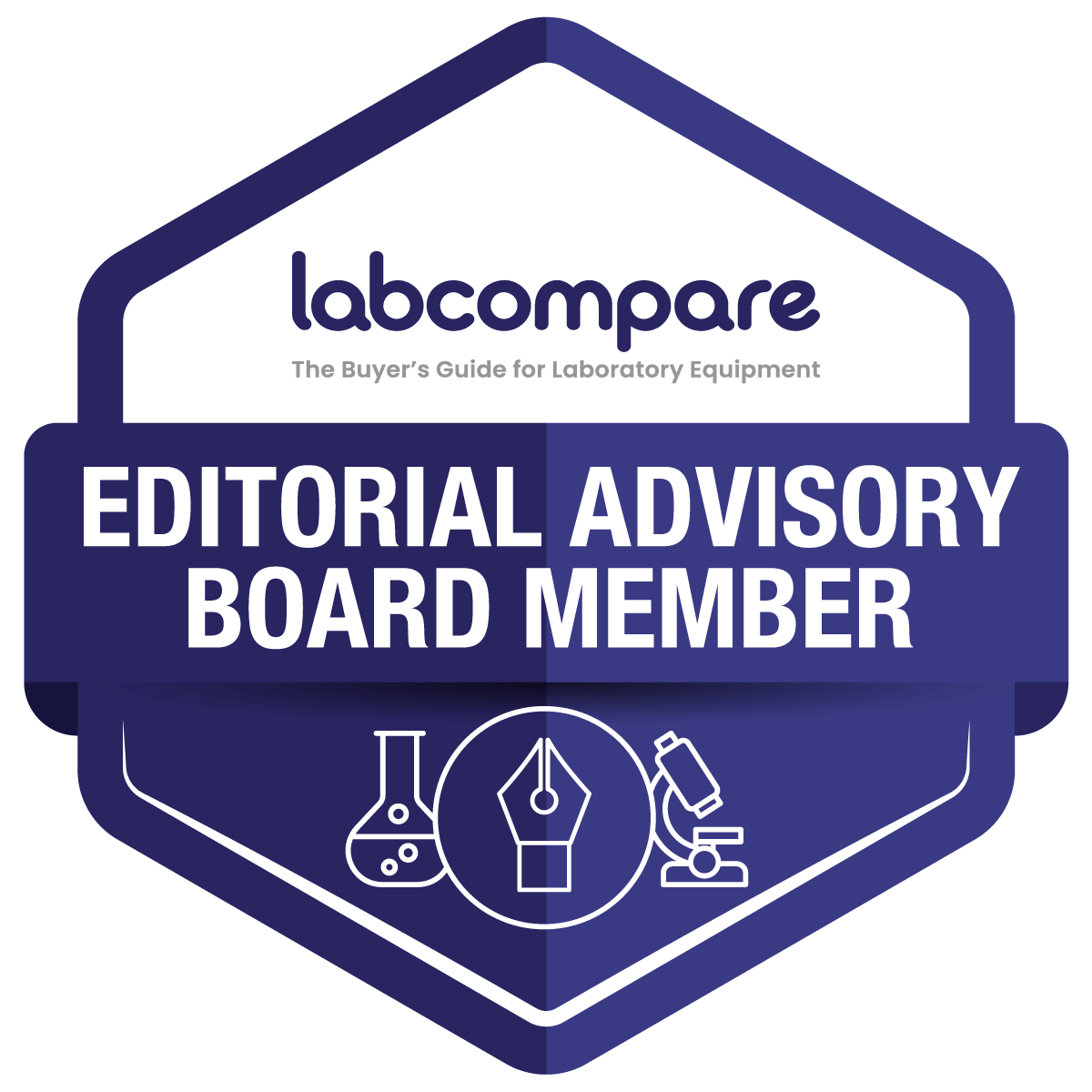
About the Author: Kevin Miller, MS, Ph.D., is the Director of Marketing and Scientific Engagement at HighRes Biosolutions and a member of Labcompare's Editorial Advisory Board. After earning a Ph.D. in Molecular Anthropology from the University of Cambridge, Miller actively engaged in promoting, researching, and furthering forensic science advances, and remains committed to this endeavor today. He developed patented software to automate forensic facial reconstruction, and served as Program Manager for a mtDNA population database for the FBI, and ultimately migrated this into the CODIS database. Miller is actively involved in forensic and crime scene associations, and publishes, lectures, and has testified on a wide breadth of forensic issues across the globe.