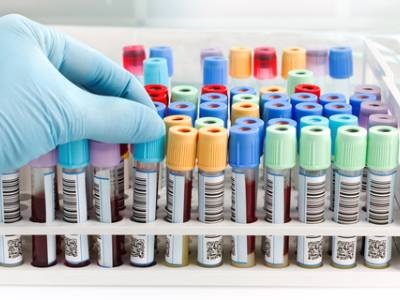
by Michael Pisano, Ph.D., Executive Vice President, Proteomics at Discovery Life Sciences
Since the dawn of the medical field, a biomarker has denoted any indicator of a patient’s medical state, like high blood pressure signifying poor cardiovascular health and risk for heart disease and stroke.1 Similarly, a protein biomarker is a molecular signal that can be indicative of a particular disease state. These protein biomarkers—often found in a patient’s blood, plasma, or tissue—can be classified as risk-factor, diagnostic, or prognostic biomarkers. Risk factor biomarkers can be used to identify patients who are likely to develop a disease, allowing for preventative treatment even before symptoms start. Diagnostic biomarkers can be used to detect early disease, classify disease subtypes, and measure responses to a therapeutic treatment. Finally, prognostic biomarkers can be used to predict disease progression, the likelihood of disease reoccurrence, and whether patients will respond to certain treatments.2 Ultimately, protein biomarkers can accelerate the drug development pipeline, shorten a patient’s diagnostic timeline, and provide insight into personalized therapeutics.3
While genomics is at the forefront of precision medicine and personalized therapeutics, given its rapid and automated workflows, there is only a weak correlation between a gene and its cognate protein. This is due to a protein’s half-life (degradation) and modifications that regulate a protein’s activity. Moreover, most therapeutic targets are proteins,4 since proteins are the active cellular machinery driving disease. Therefore, it becomes essential to view indicators of disease with a proteomics lens.
Protein Biomarker Discovery with Proteomics
Ultimately, protein biomarker discovery seeks to determine changes in the proteome under various conditions. There are two main approaches for early-phase identification of biomarkers: hypothesis-based and discovery-based.2
Hypothesis-based biomarker discovery takes advantage of mechanistic research and insight into disease progression. For example, the knowledge that elevated blood glucose levels leads to a higher percentage of glycosylated proteins was the basis for the discovery of glycated hemoglobin (A1C) as a protein biomarker for type II diabetes.4 However, since the hypothesis-based approach is targeted and relies on previous knowledge of mechanistic data, the field also utilizes discovery-based protein biomarker identification.
Discovery-based approaches rely on unbiased proteomic methods that identify protein subsets in diseased cells, animal models, or patients that differ from healthy controls. By and large, liquid chromatography with tandem mass spectrometry (LC-MS/MS) drives discovery-based biomarker identification, as this platform is entirely unbiased and assesses a large portion of the detectable proteome of a sample. In addition, since mass spectrometry delivers data down to the peptide level, it allows for the identification of variants, mutations, and post-translational modifications. LC-MS/MS analysis produces a large and complex dataset that can be used to identify hundreds of putative biomarkers when comparing proteomes of a sample from a diseased patient to that of a healthy control.5 While LC-MS/MS technology has been advancing in speed and accessibility in recent years, the dynamic range in plasma, blood, or serum limits detection with LC-MS/MS.
Challenges for the Utilization of Protein Biomarkers in Clinical Trials
The main limitation for LC-MS/MS is scalability, which poses a barrier between discovery and clinical trial work since most clinical trial samples will be blood, plasma, or serum. To overcome this barrier, companies have developed platforms for automated plasma sample prep for LC-MS/MS analysis of plasma samples. For example, Seer has developed the Proteograph™ Product Suite7 that utilizes nanoparticle partitioning of proteins to overcome the dynamic range issue presented by plasma samples allowing deep proteome coverage by LC-MS/MS analysis that is scalable.
Moving protein biomarkers from discovery and exploratory work to monitoring requires a transition from studying as many proteins as possible in small cohorts to small numbers of proteins studied in large cohorts. Once identified, verification studies will have to be performed to confirm the sensitivity of the marker(s), and ability to correctly identify a sample as positive or negative and determine if the marker(s) is/are measurable in the sample type that will be used for monitoring (e.g., plasma). This usually requires a transition from a lower throughput platform in discovery to a high throughput platform for monitoring (e.g., ELISA).
High throughput immunoassays, such as ELISA assays, are typically utilized at this point. However, multiplexing ELISA assays can be challenging, thereby limiting the number of proteins that can be detected in a single assay.8 Moreover, ELISA assays require tedious reagent (primary and secondary antibody) optimization, reducing rapid integration into clinical trial workflows. Advancements in multiplexing immunoassays using technology platforms like Luminex® Multiplex assays9 and Quanterix® Simoa® assays10 extend the breadth of immunoassays.
Ultimately, the main limitation in the transition of protein biomarkers from the discovery phase into clinical trials is the lack of scalability and rapid turnaround time. Standard protein assays used in the discovery phase, like LC-MS/MS, are challenging to implement into large patient cohorts on a strict clinical trial timeline. Therefore, most technological development in the protein biomarker field has focused on improving this scalability.
Prospects for Improved Scalability of Proteomics-based Biomarkers
In addition to the advancing speed and accessibility of LC-MS/MS-based detection of protein biomarkers, there have been significant technological advancements in antibody-based assays. For example, Olink®’s Proximity Extension Assay (PEA) technology12 employs dual antibody recognition for high specificity and DNA barcodes that are amplified by real-time PCR and read by NGS for quantitation. Unlike ELISA and other immunoassays, this platform does not have cross-reactivity limitations, enabling specific, sensitive, scalable multiplexed immunoassays that can measure thousands of proteins from a small volume of serum or plasma.
In conclusion, the limited scalability of validated detection assays has greatly limited the development and approval of protein biomarkers. However, new and innovative technologies provide a path into the future of utilizing biomarkers in clinical trial studies.
Looking Toward the Future: Integration of Transcriptomic, Genomic, and Proteomic Data with Proteogenomics
As previously mentioned, genomics is at the forefront of discovery. Genes are transcribed to messenger RNA (mRNA) and mRNA is translated to proteins. However, the strength of the correlation between a gene and a gene product (protein) can vary due to transcriptional splice variants, errors in translation, protein processing and post-translational modifications (phosphorylation, ubiquitination, glycosylation) to proteins. Therefore, there is a potential for a gene to produce multiple proteoforms. Genomics-based biomarker discovery platforms typically utilize Next-Generation Sequencing (NGS) technologies—such as amplicon, target enrichment, and whole genome sequencing—to interrogate the genomic basis of disease.
In addition, transcriptomics, another popular biomarker discovery platform, interrogates differential gene expression during disease using technologies like microarrays or RNA-sequencing. Unlike genomics, transcriptomics can detect alternatively spliced mRNAs; however, this technology still misses the final gene product, proteins and all their proteoforms.
In order to achieve a fully comprehensive picture of disease, the field of proteogenomics seeks to integrate proteomics with genomics and transcriptomics in biomarker discovery programs. By combining findings from high-throughput genomic, transcriptomic, and proteomic datasets using innovative supercomputing, the sensitivity, specificity, and precision of predicting disease-associated genes can be enhanced, adding to the biomarker discovery effort. Therefore, many leaders in the biomarker field have pivoted toward the integration of large-scale “omic” datasets.13 For example, in 2022, Discovery Life Sciences, Seer and SCIEX formed the Proteogenomics Consortium, a collaboration that will enable genomics customers to more easily add deep proteomics data to their discovery, translational and clinical studies.14
In conclusion, the field of biomarker discovery is accelerating given new technologies within the proteomics field as well as enhanced integration of large “omic” datasets.
References
- Strimbu K, Tavel JA. What are biomarkers? Curr Opin HIV AIDS. 2010;5(6):463-466. doi:10.1097/COH.0b013e32833ed177
- McDermott JE, Wang J, Mitchell H, et al. Challenges in Biomarker Discovery: Combining Expert Insights with Statistical Analysis of Complex Omics Data. Expert Opin Med Diagn. 2013;7(1):37-51. doi:10.1517/17530059.2012.718329
- Brando XC and C. The opportunities and challenges inherent in using protein biomarkers for new drug development. Drug Discovery and Development. Published December 7, 2021. Accessed October 26, 2022. https://www.drugdiscoverytrends.com/the-opportunities-and-challenges-inherent-in-using-protein-biomarkers-for-new-drug-development/#:~:text=Protein%20biomarkers%20can%20bring%20clarity
- Lyons TJ, Basu A. Biomarkers in diabetes: hemoglobin A1c, vascular and tissue markers. Transl Res. 2012;159(4):303-312. doi:10.1016/j.trsl.2012.01.009
- Tsai TH, Song E, Zhu R, et al. LC-MS/MS-based serum proteomics for identification of candidate biomarkers for hepatocellular carcinoma. Proteomics. 2015;15(13):2369-2381. doi:10.1002/pmic.201400364
- Witkowska HE, Hall SC, Fisher SJ. Breaking the Bottleneck in the Protein Biomarker Pipeline. Clinical Chemistry. 2012;58(2):321-323. doi:10.1373/clinchem.2011.175034
- Blume, J.E., Manning, W.C., Troiano, G. et al. Rapid, deep and precise profiling of the plasma proteome with multi-nanoparticle protein corona. Nat Commun. 2020; 11, 3662. doi:10.1038/s41467-020-17033-7
- Adamcova M, Šimko F. Multiplex biomarker approach to cardiovascular diseases. Acta Pharmacol Sin. 2018;39(7):1068-1072. doi:10.1038/aps.2018.29
- Luminex Platform Technology - US. www.thermofisher.com. Accessed November 1, 2022. https://www.thermofisher.com/us/en/home/life-science/antibodies/immunoassays/procartaplex-assays-luminex/features.html
- Simoa Technology. Quanterix. Accessed November 1, 2022. https://www.quanterix.com/simoa-technology/
- Leader, B., Baca, Q. & Golan, D. Protein therapeutics: a summary and pharmacological classification. Nat Rev Drug Discov 7, 21–39 (2008). doi: 10.1038/nrd2399
- Our PEA technology. Olink. Accessed October 26, 2022. https://www.olink.com/our-platform/our-pea-technology/
- Sajjad W, Rafiq M, Ali B, et al. Proteogenomics: New Emerging Technology. HAYATI Journal of Biosciences. 2016;23(3):97-100. doi:10.1016/j.hjb.2016.11.002
- Proteogenomics Consortium. Discovery Life Sciences. Accessed November 2, 2022. https://www.dls.com/proteogenomics-consortium