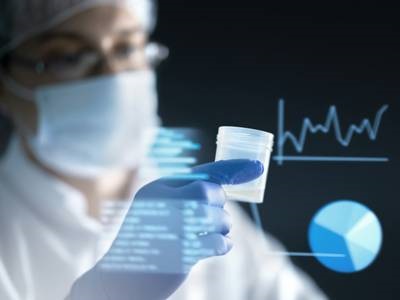
by Markus Gershater, Co-Founder of Synthace
As 2022 has come to a close, we reflect on the progress made in life sciences, but also look forward to the advancements and possibilities to come. There is an opportunity for automation to play a bigger role in overall scientific progress and for artificial intelligence (AI) and machine learning (ML) to support this. But because these technologies rely heavily on the quality and quantity of data available to them, and because the work that goes on in labs is hard to digitize and represent in code, this represents a significant challenge. Generally, we can expect to see digitization efforts continue and begin to bear fruit, but we may hopefully also see labs moving toward what we would call a post- “point solution” world. If this works, there is even potential for the barrier between wet and dry labs to finally show signs of change. Below, we’ve detailed further how these trends could impact R&D labs, the scientists that run them, and the experiments of the future.
1. Wet lab bottlenecks are here to stay unless something fundamentally changes in the way we think about how labs work.
The uncomfortable truth, though, is that the lab itself isn’t the problem. It’s the experiment. If we can’t switch to thinking about experiments as systems that can and should be digitized, and if we can’t envision or create “the experiment of the future” instead of just the “lab of the future,” we won’t see the level of change that we would hope for. With an experiment-first approach, we ask different questions about scientific value that reframe the reality of the bottlenecks we’re really dealing with.
For many, the problem of “how to improve lab automation” will remain a static issue in 2023.
Unfortunately, only a handful of early adopter R&D teams are currently asking the right questions about how automation might play a bigger role in the overall scientific progress of any given lab. Many we speak to are deeply focused on automation, but often only as an end in itself that can’t necessarily be connected to scientific value—something that is not always a factor of the volume or density of your automation.
When we talk about “value,” we’re primarily talking about the importance of understanding if experiments are feasible, reusable and reproducible—with or without automation. Can others work with and build upon my experimentation without guidance? Until we reframe the problem and instead think about the scientific throughput from an experiment-first position, traditional modes of laboratory investment will provide only diminishing returns.
2. AI/ML hype will persist in the life sciences, but only at the edges.
If many had hoped that it would start to make an impact in wet labs, they’ll find that nothing happens unless the life science industry takes a different approach to software in R&D.
AI/ML is working wonders in R&D, but its penetration into wet labs, and the data that comes from them, is nowhere close to where it needs to be. Without that data or insight, our understanding of the minutiae of lab work and how biology interacts in the real world will remain limited to the scale of individual human understanding.
Labs are notoriously hard to automate and control, and even when they are automated, the process of data aggregation and structuring is even harder. The exception to this trend will be the labs that make use of experiment platforms to design experiments, control equipment, and structure data in a single place. It’s not the lab of the future we need to worry about anymore—it’s already been here for a while—instead it’s the experiment of the future that we need to focus on next.
3. We’re moving to a post-point solution world; those that don't move with the times will be left behind.
After decades of focus on, and investment in, software that addresses specific digital problems in labs, this approach now makes diminishing returns. In the new world, experiment platforms will win out. They open labs up to possibilities that point solutions will never be able to realize.
Digital “point solutions”—such as electronic lab notebooks (ELNs)—have long been the only way to digitalize lab work. While these tools are individually sophisticated, they are still only a collection of parts that fail to make a convincing sum.
R&D is desperately in need of a new era of digital tools with functionality that cuts across all these individual point solutions. They won’t combine and unify all the same functionality or even necessarily replace them, but they will reach into and improve their functionality, making it easier to understand and measure scientific value—all while opening involvement in the process of lab work to a larger population of people who work primarily in the “dry lab,” such as bioinformaticians.
4. After years of frustration, the barrier between wet and dry labs has the potential to finally show signs of change.
The main reason behind this is the potential for innovative software solutions that address these barriers directly, closing the loop between what goes on in wet labs and the enormous value bioinformaticians and data scientists can bring to experimentation.
Taking an experiment systems approach helps bioinformatics and data scientists as well. Too often the data analyzed outside of the lab has no way to loop back into new experiments because it often relies on intense and laborious manual intervention. That is, it relies on the heavy lifting of individuals to translate the different various mental models of the experiment so that analysis can be used to inform the next round of work in the lab.
With a shared mental model of the experiment held on a digital platform, this becomes significantly easier.
5. “Lab software” will only get us so far in life science R&D.
It fixes specific problems really well, but it can’t guarantee that it will improve the fundamental building block of scientific understanding: the experiment. The future? It's experiment software or bust.
For years, better equipment and better software have helped biologists make incredible advances. But, today, the only thing that better lab software guarantees is better lab software – it doesn’t mean better experiments. Unlike the endless stream of lab software that has long come before it, experiment software makes experiments more powerful, more scientifically valuable, and easier to run. It doesn’t replace ELNs, laboratory information management systems (LIMS), or data systems (although, long term, those things may become less useful or even redundant). Experiment software will instead make all of those things more valuable than before: the data, metadata, and contextual experiment information that flows from experiment software are far more valuable, accurate, and rapid than anything that relies on manual input and intervention.
Of all of the trends in our industry, this one will be the most impactful, as the experiment is the most fundamental foundation of scientific progress. If our industry succeeds in making this happen, experiments stand to become more powerful than we ever might have imagined, and a new world of possibility will open up.