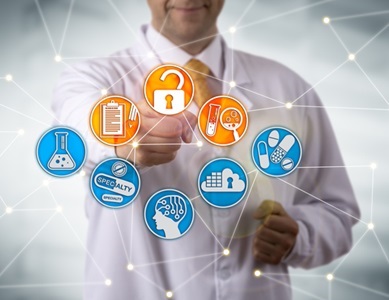
by Rafael Rosengarten, Ph.D., Chief Executive Officer and Co-founder, Genialis
Over two decades ago, the publication of the first human genome ushered in a wave of hype and hope about the promise of genomic-informed medicines. With all the genes becoming knowable, the cause of all diseases could be known too. Of course, the one-gene-one-disease model did not hold up in reality (in most cases). Nevertheless, over the years researchers have been able to assign a disease-causing role for numerous mutations, including those in prominent cancer driving genes such as BRCA1/2, EGFR, HER2 and KRAS, to name a few. Sure enough, genome-targeted and genome-informed therapies became a reality.
Drugs discovered against these targets are intended for precise activity at the source of the disease. Over the years, the number of patients eligible for genome-targeted and informed therapies has climbed slowly, from 10.7% of all cancer diagnoses in 2006 to 27.3% in 20201. This increase represents both a greater number of drugs, but also improvements in the availability of diagnostic biomarkers. Meanwhile, in the same time span, the response rate to these therapies has increased modestly, from 3.3% to 11.1% of cancer patients. The durability of response, however, remains statistically unchanged, at 18.9 months and 17.6 months over those 14 years. Yes, the eligibility and overall response to genomic medicines for cancer have gone up, but to many in the field, as well as to patients and their families, these numbers remain woefully inadequate.
One solution to increase both the number of patients eligible for genome-informed therapies, but also the effective response rate, are predictive biomarkers. A biomarker is any biological measurement that can be used to infer the status of one’s health or disease. A predictive biomarker is a biological measurement that provides a probability of response to a therapeutic intervention (i.e. clinical benefit to a drug). Predictive biomarkers can be used to pre-select patients for clinical trial enrollment, or if developed into a diagnostic test, to guide or inform therapeutic decision making. Examples include HER2 levels in breast cancer2 and the use of Trastuzumab in HER2-positive breast cancer3, or assessment of EGFR T790M mutations in metastatic NSCLC, which guides the use of Osimertinib when patients are resistant to first-generation tyrosine kinase inhibitors like Erlotinib4. A recent retrospective study of the top 5 cancer indications showed that a clinical trial was 5-12x more likely to meet its endpoint if that trial included a biomarker5. Thus, biomarkers can impact real world medical decision making, and increase the odds of promising new drugs to gain approval and reach the market.
To truly help close the gaps in eligibility and response to genome-informed therapy, in ensuring that most cancer patients have the opportunity to receive the best possible precision medicine, new kinds of biomarkers are required that capture more biological information than previous generations. Today the 150 or so FDA approved companion diagnostics represent roughly 30 unique biomarkers, and most of these are single analytes—e.g. one protein or one genomic alteration. For complex diseases such as cancer, one cannot hope to understand the state of the disease from a single measurement. Instead, new biomarkers are emerging that take advantage of decreasing sequencing costs and more robust laboratory methods to incorporate entire genomic spectra of mutations, transcriptome wide gene-expression patterns, or dozens to hundreds of proteins and post-translational modifications, all at once. These high-dimensional analytes provide much more information, and in the case of RNA and proteins, are representative of the dynamic physiological state of the disease, and thus can report on the druggable phenotype.
Such vast information creates new challenges, however, including the requirement that one can define a clinically tractable signature that can be reliably and reproducibly measured from available tissue at a reasonable cost. Machine learning (ML) can be an ideal tool to identify and define such a signature. ML is a branch of artificial intelligence, a mathematical tool kit consisting of various kinds of algorithms that share a key characteristic of being good at detecting patterns from large amounts of data. Machine learning can be applied to genomic, transcriptomic and/or proteomic data (not to mention imaging data from pathology slides or other scans), to help discover signatures and develop these into diagnostic models. Sure enough, the use of AI in FDA cleared devices is rising rapidly.6
Employing AI/ML as the algorithmic engine for a clinical biomarker does not change the requirement that the biomarker be reliable, reproducible and interpretable. Meanwhile, these technologies can exacerbate one area of risk when developing diagnostic tools—bias. Bias refers to the fact that every data set not only provides information about the biology, health or history of the patient, but also reflects the conditions, decisions and mechanics by which the data was collected. For example, the overall healthcare data available in the world has been shown to harbor severe gender bias, in which female patients are grossly under-represented7. Similarly, healthcare data tends to underrepresent people of color, socioeconomically disadvantaged individuals, and less economically developed nations. In addition to these systematic biases, molecular data like RNA-sequencing will have biases related to patient ethnicity, tissue type, tissue handling, sequencing method, sequencing platform, etc.
Source
|
Bias type
|
Healthcare equity, access
|
Collection bias
|
Geography, ethnography
|
Genetic, lifestyle bias
|
Technology
|
Chemistry, platform bias
|
Biology
|
Tissue, disease heterogeneity bias
|
Thus, in developing ML models as clinical biomarkers, addressing bias is a requirement. Any model trained on biased data will fail to perform in clinical contexts that vary from that of the training data, and thus will not serve patients in the real world. ML itself, however, can be an important tool in combating bias. Algorithms can be trained and built into quality control (QC) workflows to identify biased or imbalanced datasets. For example, classifiers can detect gender gaps in the data, quantify biological biases such as tumor versus normal, and evaluate the differences between globally sourced data. Prior to training a diagnostic ML algorithm, the subset of genes or proteins that feed into the analysis can be optimized to minimize the amount of bias detected in that feature set. QC algorithms can also be used to harmonize data to make disparate sources interoperable. Assembling training data across axes of bias can lead to models that outperform single-source models in the real world because they have learned clinically relevant signals as a common denominator.
Thanks to novel approaches in the application of AI/ML, with more robust selection of features and automating QC before model construction, it is possible to identify and circumvent biases in existing data. Detecting and addressing data biases is enabling a class of biomarkers that use high dimensional data to represent complex disease biologies. These biomarker models can be trained to learn fundamental disease biology, rather than being trained directly on clinical outcomes. Further, curated datasets that are more representative of the relevant patient population across the globe yield models that have more broad clinical utility. These next generation biomarkers will be a game changer in terms of reliability and adaptability across demographically distinct cohorts and may help begin to close some of the gaps in access to precision medicine that now exist.
References
1. Haslam, Kim & Prasad, Annals of Oncology, 2021
2. Burstein HJ. The distinctive nature of HER2-positive breast cancers. The New England Journal of Medicine. 2005;353(16):1652–1654
3. Hudis CA. Trastuzumab—mechanism of action and use in clinical practice. The New England Journal of Medicine. 2007;357(1):39–51
4. www.nccn.org/professionals/physician_gls/pdf/nscl.pdf
5. Parker et al., Cancer Medicine, 2021.
6. https://www.fda.gov/medical-devices/software-medical-device-samd/
artificial-intelligence-and-machine-learning-aiml-enabled-medical-devices
7. Invisible Women