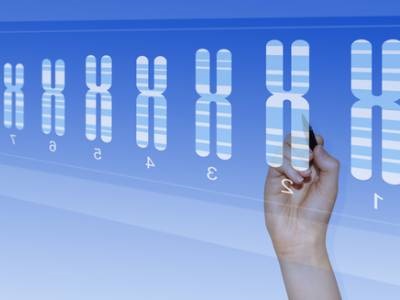
By John Burrill, Product Management Director, Thermo Fisher Scientific
Through the years, advancements in cytogenetics research have improved our understanding of the origins of a variety of genetic diseases and some types of cancer. Recent technological advances in genetic analysis have led to the introduction of high-resolution, genome-wide techniques for chromosomal analysis, such as chromosomal microarray analysis (CMA), exome sequencing (ES), and genome sequencing (GS). With the increased utility of these test methods in clinical research studies, lab directors and clinical scientists face the challenge of determining when to use which method for the best results.
Advancements in genetic analysis techniques
Conventional cytogenetic workflows like karyotyping, fluorescence in situ hybridization (FISH), and different polymerase chain reaction (PCR) based tests have been a part of the genetic analysis industry and instrumental in identifying major chromosomal abnormalities. However, these conventional techniques have limitations when it comes to chromosomal analysis in that they can only identify a certain subset of genetic aberrations and often only find the precise faults sought, and those with low resolution can only identify larger aberrations.
Compared to conventional techniques, advanced approaches like CMA, ES, and GS can provide more thorough data about a person's entire genome that can help with the identification of genetic abnormalities.
Chromosomal Microarray Analysis: Chromosomal microarray analysis (CMA) is recommended to gain deeper insights in genetic analysis. Researchers used to rely on microscopes for chromosomal analysis before the advent of CMA, which is now seen as a tried-and-true genetic analysis method. The research tool is designed to look for copy number aberrations and determine if there are chromosomal imbalances; either large or smaller extra (micro-duplication) or missing (micro-deletion) pieces of genetic information, also called copy number variants (CNV). Micro-duplications or micro-deletions may be associated with significant health and/or developmental problems despite their small size. Fortunately, CMA can identify chromosomal aneuploidy and other large changes in the structure of chromosomes as well as submicroscopic abnormalities that are too small to be discovered by traditional modalities.
Recent innovations in CMA are helping address one of the main challenges cytogeneticists face: data analysis and interpretation. Integration with digital automation is contributing to greater lab efficiency by streamlining data analysis and interpretation, making a process that used to take hours almost immediate. With a massive amount of data at their fingertips, new analysis tools can help researchers quickly prioritize the most important mutations in a sample, greatly increasing productivity for lab directors and clinical geneticists by minimizing time spent manually searching for genetic associations. In addition, innovations in array technology are enabling high resolution copy number analysis, reduced need for sample culturing, fewer inconclusive results, and faster turnaround times. Moving forward, microarrays will remain a platform of choice for efficient, cost-effective high-content genetic research.
Exome sequencing: Next generation sequencing (NGS) is increasingly being used in genetic analysis to boost discovery yield and improve outcomes. Used to study many genetic disorders, exome sequencing (ES) is a widely used NGS-based method that involves sequencing the protein-coding regions of the genome, known as exons, that represent about 1.5% of the entire human genome. Since most disease-associated genetic variants occur in the exome, it is efficient to focus the search specifically on this part of the genome, in turn making it a much faster and less expensive research method than genome sequencing to identify disease associated genetic variants or confirm a clinical observation.
ES limitations include the risk of missing variants in the non-coding regions, challenges analyzing larger DNA structural variations, trinucleotide report expansions, and methylation anomalies, and limited capabilities for identifying CNVs. In addition, unlike with CMA, ES lacks standardization. There are many different calling techniques available, but there is no agreement or guidance on how to use those techniques. In fact, due to the lack of comprehensive guidelines for use of ES-based CNV discovery tools for studies in clinical research settings, research teams have conducted several studies to compare these techniques. One of the studies found that using multiple techniques in combination obtained the best balance of sensitivity and practicality. However, this approach increases cost and turnaround time.1
Genome Sequencing: Genome sequencing (GS) analyzes the entire genome – 3.2 billion base pairs – to generate a vast amount of data. Although obtaining more data may seem desirable, when it comes to cytogenetic analysis, less data can be “more.” In some cases, more data increases the likelihood of identifying variants of uncertain significance and secondary findings, and in many cases, without finding the causal variant. All of these instances can complicate data interpretation and reporting, thus requiring expert-level data analysis by a qualified bioinformatician. Therefore, when conducting routine constitutional genetic analysis, GS is not advised. However, GS may be considered for rare genetic disorders when no other test methods have yielded a result. For the most part, GS takes significantly longer to perform, requires more data storage, has a more complex analysis process, and is more expensive than ES and CMA.
Recommendations from professional societies
As clinical and scientific advances continue to increase the applications for genetic analysis technology in clinical research labs, determining which genetic analysis method is most suitable for specific applications can help improve research outcomes. It is important for scientists to keep up with the recommendations and guidelines issued by various professional societies and their recommendations for the use of CMA, ES and GS.
Professional societies such as the American College of Obstetricians and Gynecologists (ACOG), American Academy of Pediatrics (AAP), American Academy of Neurology (AAN), American College of Medical Genetics (ACMG), and the European Society of Human Genetics (ESHG) have provided guidelines and recommendations for constitutional cytogenomic analysis. For the most part, these societies agree and recommend prenatal chromosomal microarray analysis for research studies of individuals with intellectual disability, autism, neurodevelopmental disorders, and/or congenital anomalies due to its high discovery yield.2It is also recommended that CMA be performed in research studies when a fetus with one or more major structural abnormalities is identified with ultrasonographic examination. As for ES and GS, the professional societies for the most part agree that they should be used as a second-tier analysis method after performing CMA because of higher results obtained with lower cost.
Factors to consider when choosing a analysis technique
When choosing a genetic analysis platform for research studies, lab directors and clinical scientists must take into account operational factors, such as data quality, resources needed for genetic analysis, turnaround time, and cost, in addition to understanding the analytical capabilities and limitations of each technology and professional societies' recommendations.
Data quality is affected not only by the primary data generated by the researcher but also by the analysis, interpretation, and reporting. To generate quality data, labs need to consider ease of use and interpretation, as well as the flexibility to produce custom reports. For CNV analysis, CMA has more robust, out-of-the-box, standardized analysis tools. Another way research labs can generate quality data is to adhere to best practices and technical standards provided by professional societies.
As important as data quality is for a lab, so is turnaround time (TAT). One way to measure the efficiency and productivity of a lab is by considering the time it takes between sending a sample to the lab and receiving the results. TAT is very important, especially in prenatal research studies and in some cases, postnatal research. Therefore, when selecting a genetic analysis method, labs should consider assay time, including hands-on time, as well as data analysis, interpretation, and confirmatory analysis time.
Labs should also consider resources required to support analysis, including space for instruments and workbenches. Importantly, the budget for implementing an end-to-end solution can vary widely. This includes funds for confirmatory testing, computational requirements, IT staff, data storage and backup, instrument maintenance and service, and qualified personnel. These resources should be scalable to handle rising sample quantities. As an established and user-friendly option, CMA doesn’t have the same requirements for expert-level bioinformatics, and generally does not require large instruments or expensive computational equipment for data analysis and storage.
Finally, the cost of running analysis must also be considered. In general, the cost per sample depends on throughput. Higher throughput, in principle, lowers the cost per sample. For CMA, the cost per sample is less dependent on the number of samples processed per run than with NGS-based methods. In order for NGS to obtain a cost per sample similar to that of CMA, a high throughput instrument with high volume runs is needed.
The right tool for the job
Choosing the right genetic analysis method can drastically improve outcomes. CMA, ES, and GS are powerful genome-wide chromosomal aberration analysis tools for constitutional genetic research. Whether selecting CMA or NGS, cytogeneticists should keep in mind that each method has its technical and operational advantages and limitations.
NGS technology is transforming disciplines in life sciences, but the technology is still evolving for genetic analysis. Therefore, it is advised that researchers stay up to date on professional society guidelines and recommendations.
CMA is more established, robust, and requires fewer resources, including bioinformatics, to implement than NGS. Fortunately for labs, CMA continues to evolve. Advances in microarray technology and data analysis software will greatly accelerate turnaround times and interpretation, making CMA a cost-effective and reliable choice for labs looking to increase throughput for prenatal and postnatal research.
There is no “one size fits all” method. The chosen analysis strategy should consider TAT, cost, and the best outcome for the end user.
About the Author: John Burrill is Director of Product Management and Market Development, Microarray Reproductive Health.
John was Director for Software strategy, Reproductive Health before taking on the role of Director for Product Management and Market development for Reproductive Health in 2020. John joined Thermo Fisher Scientific as Director of Portfolio and Program Management in March 2016 through the acquisition of Affymetrix. Previously at Affymetrix, John served in a variety of roles in the Software Development organization, culminating in being Sr. Director for Software Development leading the team responsible for Affymetrix commercial software. Prior to joining Affymetrix, John worked at Applied BioSystems and Incyte Genomics.
John is a graduate of the University of Chicago and holds a Ph.D. in Neuroscience from the University of Michigan.