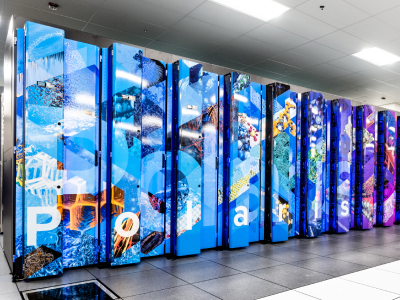
A photo of the Polaris supercomputer. Courtesy of Argonne National Laboratory.
Researchers from the University of Michigan are developing an atom-predicting AI tool with support from the Argonne National Laboratory. The model will have a focus on small organic molecules relevant to energy storage applications.
Supported by a one-year Department of Energy grant, the researchers will have access to 200,000 node hours on the Argonne National Laboratory 34-petaflop supercomputer called Polaris. With a focus on energy storage and conversion, the model will primarily focus on carbon, hydrogen, oxygen, and nitrogen.
"What we've learned from language models is that size matters. The interesting behavior happens when you make it very big," said Venkat Viswanathan, University of Michigan associate professor of aerospace engineering. "If you train on a small amount of data and ask it to write Shakespeare, it's not good. But a larger data set is better, and when it's big enough, text that sounds like Shakespeare emerges."
The team plans to train the model for use in predicting battery electrolytes. With the promise of increased energy density over current lithium batteries, the researchers believe that AI can assist in discovering electrolytes that can work with alternative electrode pairs. The model will be fed text-based representations of atomic structures without any additional information.
"There are several billions of molecules that are possible to make, and we have the text-based representation for them," said Viswanathan. "Our slice of that will be synthesizable small molecules similar to those used in pharmaceuticals and electrolytes."
Once the model is functional, the team will ask it to predict electrolytes that are suitable for a particular pair of electrodes. This information will then be used to test each configuration using a robotic laboratory setup. The researchers are hopeful that AI can identify new design rules that have not yet been considered. "When we learn chemistry, we learn each rule, and then we learn about a dozen exceptions," said Viswanathan. "Can this now help us learn better rules or be able to design with more sophisticated combined rules?"