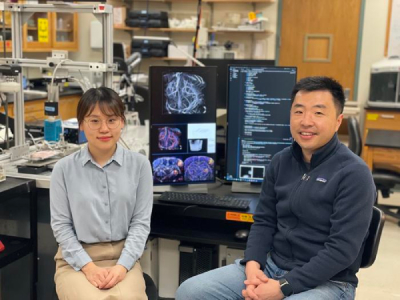
YiRang Shin (left) and Pengfei Song in the Song Lab at the Beckman Institute for Advanced Science and Technology. Credit: Elizabeth Bello, Beckman Institute Communications Office.
Beckman Institute researchers have developed a new microscopy technique to improve high-resolution microvascular imaging. The method utilizes deep learning to aid in post-processing, making the method more practical for use in a clinical setting.
Ultrasound localization microscopy, or ULM, is an emerging method being used for the high-resolution imaging of microvascular systems. Currently, the technique has limited use as a diagnostic tool because of the difficult post-processing required. The novel technique, published in Nature Communications, called LOcalization with Context Awareness Ultrasound Localization Microscopy, or LOCA-ULM, improves the sensitivity and performance of the technique while reducing the time required for processing.
“I’m really excited about making ULM faster and better so that more people will be able to use this technology. I think deep learning-based computational imaging tools will continue to play a major role in pushing the spatial and temporal resolution limits of ULM,” said YiRang Shin a graduate student in the Department of Electrical and Computer Engineering at the University of Illinois Urbana-Champaign.
Ultrasound localization microscopy relies on the injection of microbubbles into blood vessels to act as contrast agents. Once injected, ultrasound waves can penetrate tissue and pinpoint the location of the microbubbles as they travel. This information can be used to track blood flow speeds and to create microscale spatial images of blood vessels in the human body. Previous methods to increase the imaging speed capabilities of the technique required higher concentrations of these microbubbles, an addition that also caused an increase in the post-processing required.
The method developed by the researchers improves localization performance while being adaptable to varying concentrations of microbubbles. The method relies on a novel generative adversarial network to generate simulations to train a deep context-aware neural network called DECODE. “It really beats conventional microbubble localization methods; this is the way to go,” said Pengfei Song, assistant professor of electrical and computer engineering at Illinois.